This fall, a virtual gathering on machine learning and statistical methods will sketch out related projects within ARM and ASR
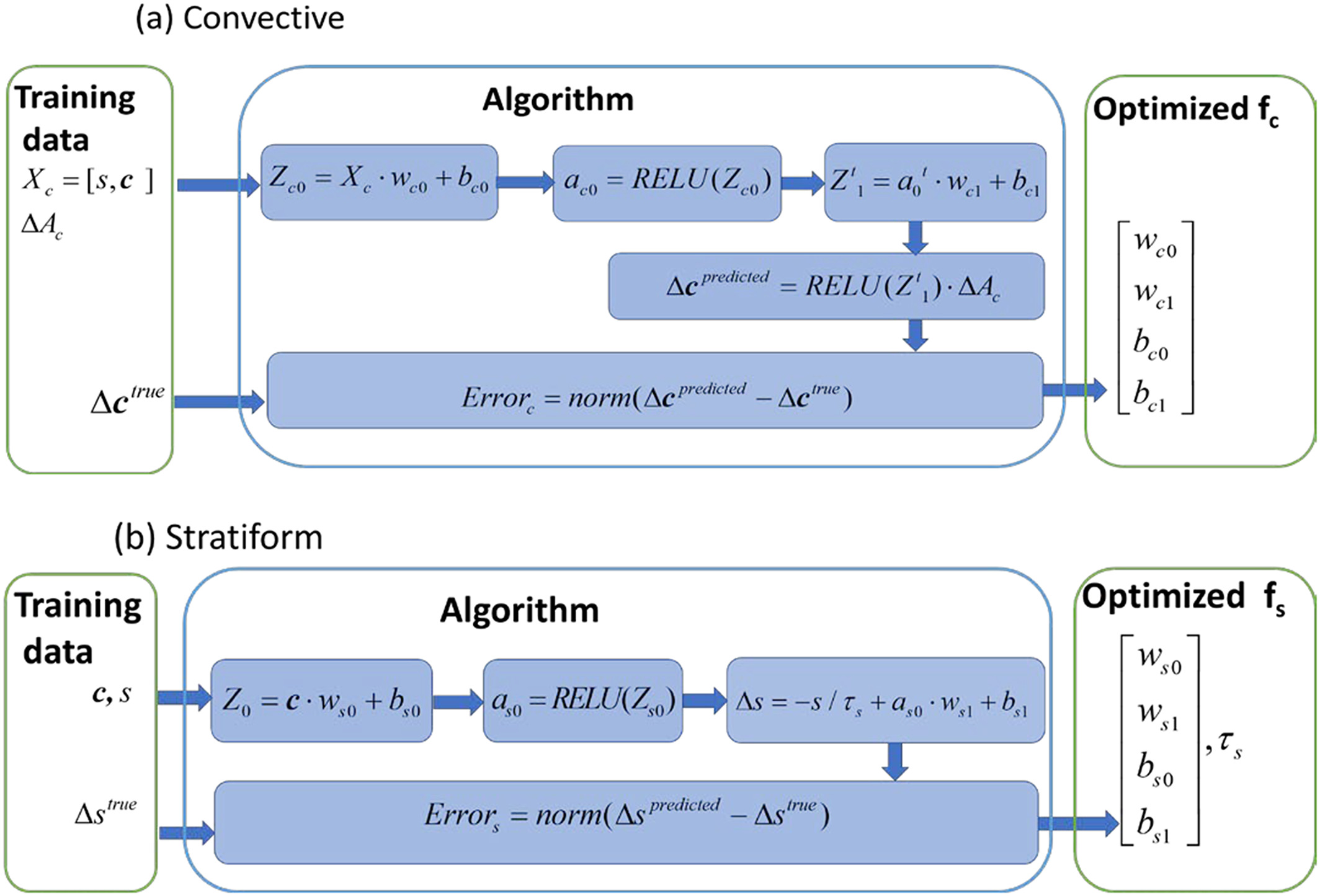
Machine learning (ML) is an application of artificial intelligence (AI). Developers create and test a wide range of algorithms that help computers sift through data in search of inferences. Once set in motion, ML requires no explicit programming. In time, computers begin to learn from data.
Because ML automates analysis, it is useful in speeding the computational efficiency of systems used in a variety of critical fields, including finance, health care, retail, infrastructure, and transportation.
ML has the potential to revolutionize science, including the atmospheric sciences. Researchers are increasingly overwhelmed with big data sets that require unlocking and analysis. To help, ML finds correlations and predictors in data that would otherwise remain hidden or require intensive human labor to optimize.
To acknowledge and further the power of such approaches, interested researchers are invited to attend a virtual workshop on October 19 and 20, 2020. From noon to 4 p.m. Eastern time each day, presentations and discussions will unfold regarding ML and other statistical methods related to observations and modeling.
The October workshop takes the place of breakout sessions that normally would have happened at the 2020 Joint Atmospheric Radiation Measurement (ARM) User Facility/Atmospheric System Research (ASR) Principal Investigators Meeting, which took place virtually in June.
The Shape of Things
A call for workshop speakers wrapped up in September, and the agenda is available now. Topic areas include:
- updates from the U.S. Department of Energy’s July 2019 AI for Science town halls
- applications of ML, AI, and deep learning to atmospheric science observational and modeling problems
- applications of a variety of statistical methods that use observations to reduce model uncertainty, including ML, data assimilation, and inverse modeling
- novel applications of ML to ARM data
- improving ARM and ASR accessibility to ML solutions
- immediate research needs that could benefit from ML.
Workshop organizers are Susannah Burrows, Jennifer Comstock, Joseph Hardin, Rao Kotamarthi, Jitendra Kumar, Nicole Riemer, Manish Shrivastava, Adam Theisen, and Marcus van Lier-Walqui.
Invited speakers include climate-data scientist Libby Barnes of Colorado State University and Amy McGovern of the University of Oklahoma.
McGovern directs the new AI Institute for Research on Trustworthy AI in Weather, Climate, and Coastal Oceanography, funded by the National Science Foundation.
# # #ARM is a DOE Office of Science user facility operated by nine DOE national laboratories.