Using ARM Data, Scientist Helps Bridge Gap Between Observations and Global Modeling
Published: 19 August 2019
Maike Ahlgrimm leaves the European Centre for Medium-Range Weather Forecasts as a key contributor to its longtime relationship with ARM
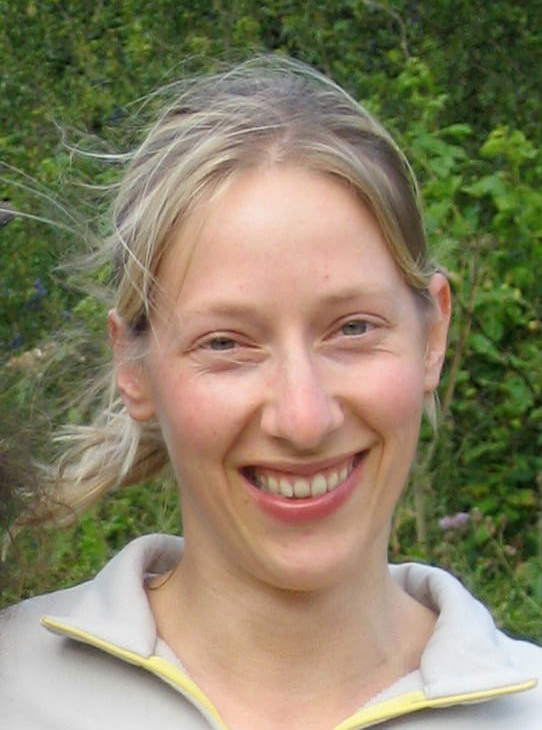
In a partnership of more than two decades, data from the Atmospheric Radiation Measurement (ARM) user facility helped to evaluate and improve global modeling at the European Centre for Medium-Range Weather Forecasts (ECMWF).
Several scientists at ECMWF, including Maike Ahlgrimm, have worked directly with ARM data over the years to improve understanding and modeling of the atmosphere. Since 2008, Ahlgrimm has spent the bulk of her career digging into data from ARM’s observatories around the world to continue improving the ECMWF model, which typically produces forecasts up to two weeks ahead.
“Over the last number of years, Maike’s been key at that relationship, not only to work with that particular model, but to provide a window into the global modeling community in general,” says ARM Technical Director Jim Mather.
Now Ahlgrimm is embarking on a new phase of her career. After leaving the England-based ECMWF in July 2019, the Berlin native has started work with the German weather service.
With Ahlgrimm’s departure, the active research collaboration between ECMWF and ARM is over for the time being. However, a mutually beneficial data exchange between the two institutions will continue, and a wealth of important achievements resulting from the research collaboration will live on. This includes ECMWF supporting ARM with forecast and analysis data for its fixed and mobile atmospheric observatories.
One-Stop Shop
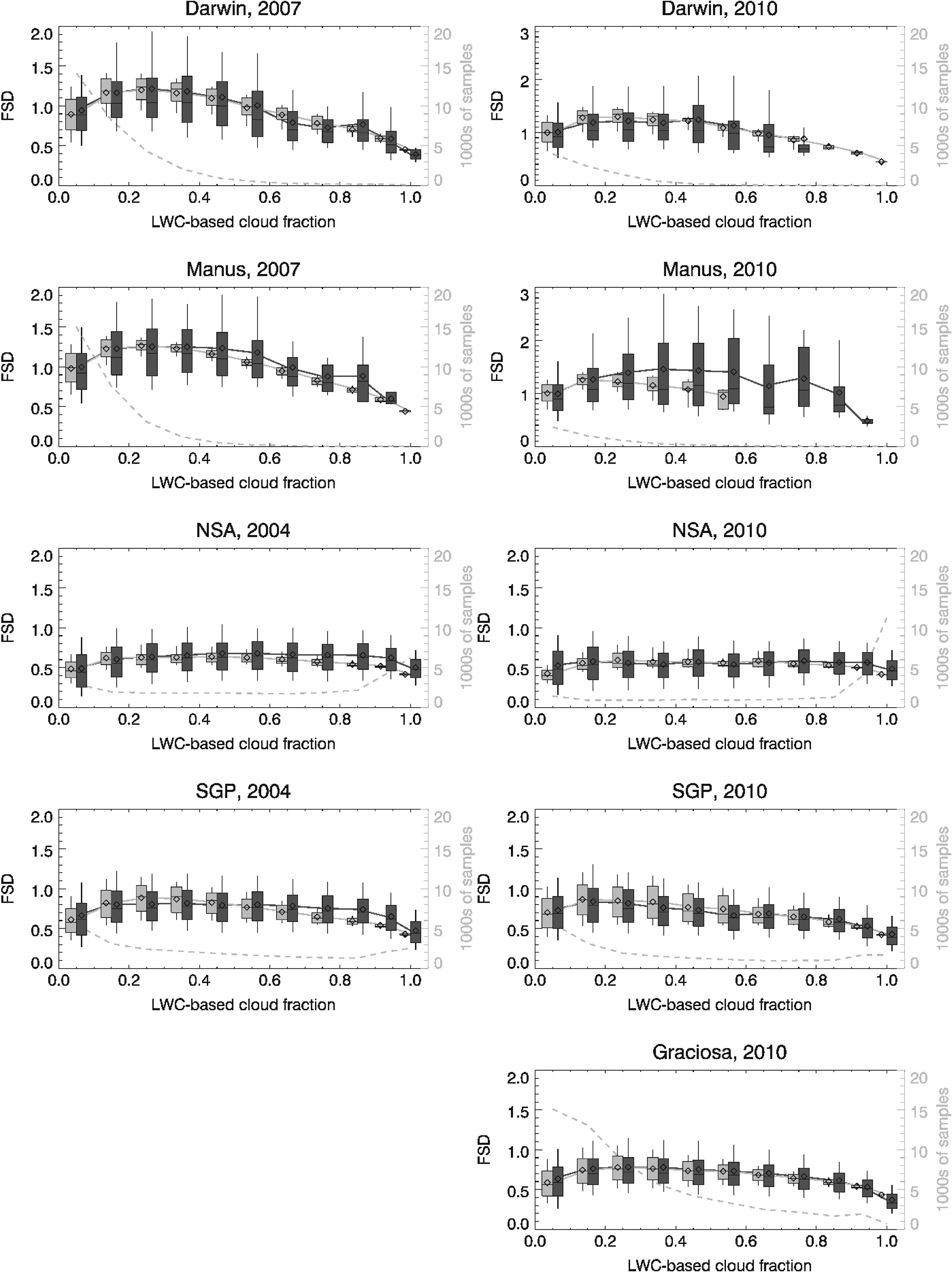
Ahlgrimm has gotten intimately familiar with both the ECMWF model and ARM observations, including value-added products (VAPs) that deliver comparable data from a variety of locations.
“Whenever you want to make changes to a global model, you really have to get to know the guts of the model as well as the observations,” says Ahlgrimm, who received funding from the U.S. Department of Energy’s Atmospheric System Research program in recent years. “With grants typically covering a three-year period, we have covered a range of science areas to address hot topics for global modeling and make best use of new observations and data products.”
In 2016 and 2017, the Quarterly Journal of the Royal Meteorological Society published papers by Ahlgrimm and ECMWF colleague Richard Forbes that explored the variability of cloud water, or condensate, over multiple ARM sites. Data came from ARM’s Southern Great Plains, North Slope of Alaska, and Tropical Western Pacific observatories, and from the eventual home of ARM’s Eastern North Atlantic (ENA) observatory in the Azores west of Portugal.
Ahlgrimm found that condensate varied more in liquid clouds over the tropical sites than in those over the midlatitude or arctic sites. In response, she modified a parameterization to put into the ECMWF model. Compared with ARM data, the parameterization captured how condensate variability changed with climatic region, cloud type, and season.
Looking at ice clouds using ground-based data proved to be more difficult.
“The ice clouds we can see from the ground tend to be only the stand-alone cirrus clouds or the anvil outflow,” says Ahlgrimm. “Anytime you have a really deep convective system, the ice cloud above is completely blocked by the precipitation and warm cloud underneath.”
Following the idea that if you want to look at a high cloud, you should look at it from the top, Ahlgrimm developed a parameterization for condensate variability in ice clouds using satellite observations. She then checked the parameterization against ARM observations, and the ground and satellite data agreed well.
Narrow Focus, Wide View
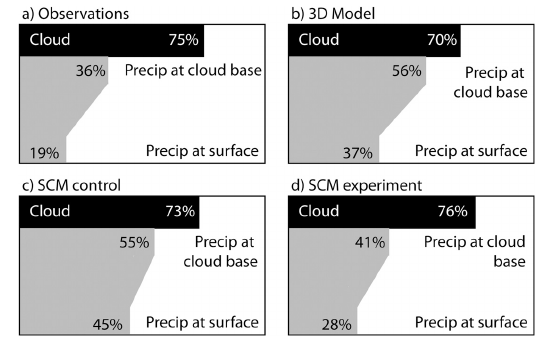
Information from an individual site can be trickier—though not impossible—to incorporate into the ECMWF model.
“For me, one of the greatest challenges has always been to take observations from a single site to something that’s relevant to a global model, because whatever you put into the model based on observations from that single site has to be applicable globally,” says Ahlgrimm.
Ahlgrimm and Forbes looked at data from ARM’s North Slope of Alaska observatory to develop a more realistic method of representing supercooled liquid water layers in arctic mixed-phase clouds, which are composed of liquid and ice. The new representation, which appeared in a 2014 Monthly Weather Review paper, incorporated separate liquid and ice variables instead of a mixed phase that depended on temperature.
In another 2014 paper published by Monthly Weather Review, Ahlgrimm and Forbes compared modeled drizzle with ARM data from the Clouds, Aerosol, and Precipitation in the Marine Boundary Layer (CAP-MBL) field campaign in the Azores. The mobile campaign deployment site, Graciosa Island, is now home to ARM’s fixed ENA observatory.
The authors noted that the ECMWF model overestimated the occurrence of drizzle at the cloud base and at the surface. They concluded that improving the treatment of warm-rain formation would resolve much of the drizzle discrepancy at the cloud base.
Ahlgrimm is working on another paper about drizzle using ENA data. The ECMWF parameterization for evaporation was coming up short because it was only considering the mass of the drizzle. Ahlgrimm believes the model also needs to predict the number of droplets to avoid missing the smallest drops.
A Unique Voice
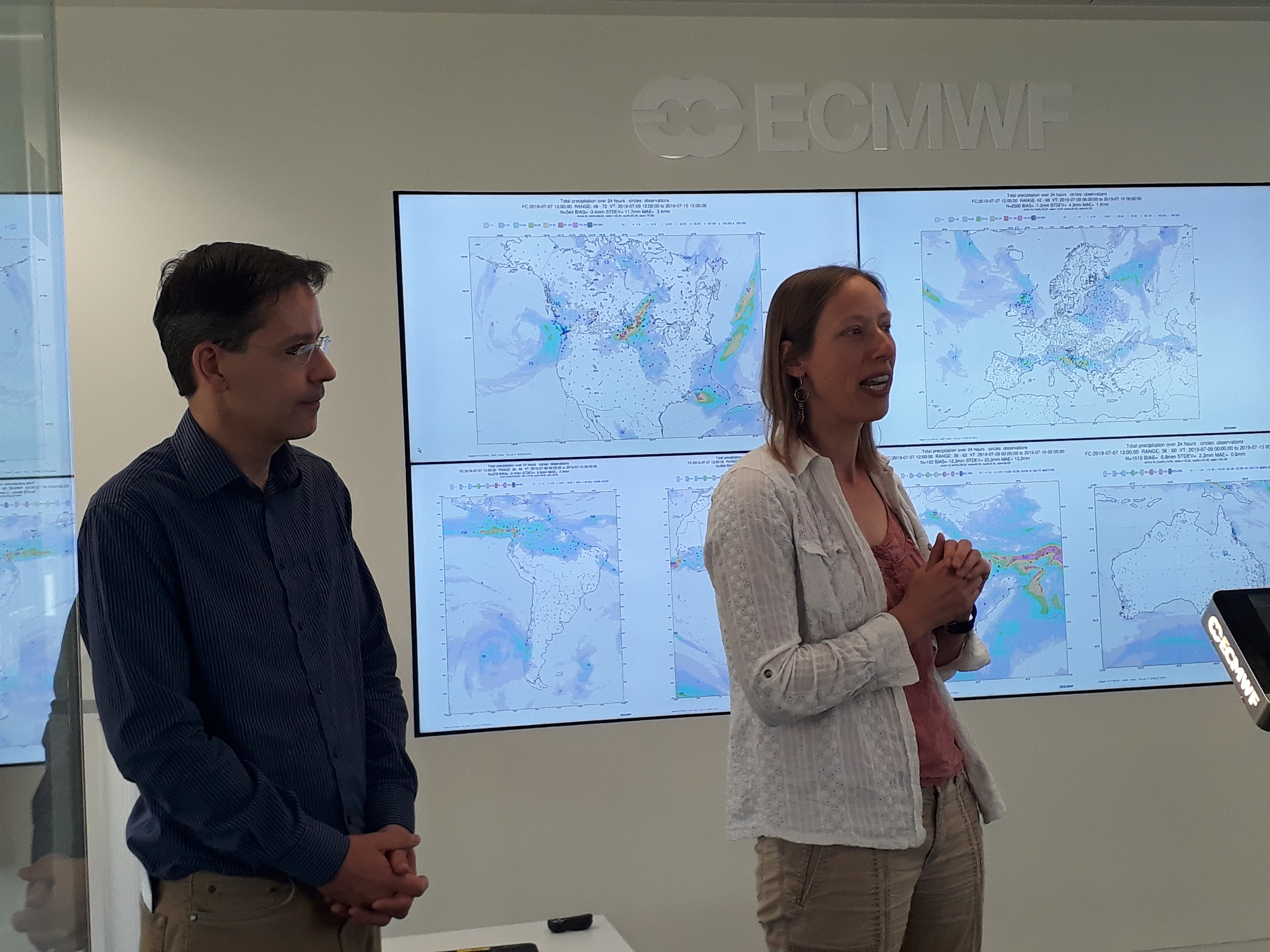
Ahlgrimm has been an active, engaged member of the ARM user community. In 2016, she was the corresponding author of a chapter on the ECMWF-ARM research collaboration in the ARM monograph, which chronicled the first 20 years of the U.S. Department of Energy scientific user facility.
She has also attended the annual Joint ARM User Facility/Atmospheric System Research Principal Investigators Meeting, presented at ARM workshops, and served on the ARM Atmospheric Modeling Advisory Group, providing valuable insights into what global modelers want and need from data products.
“It’s unusual to have a modeler of that sort that’s really digging into the observations in that way,” says Mather.
Ahlgrimm notes that ARM has done a great job addressing her feedback.
“The archive has come a long way over the past 10 years, and some of the VAPs have become much more accessible,” she says. “One of the things that I’ve been pointing out for years is the timeframe—how long it takes from taking the measurement to actually having the VAPs in the archive—and I think they’ve really worked on that as well.”
Ahlgrimm acknowledges that her perspective is rare. It is common for people to work with observations or models, but not both.
“It’s a really wide gap between going out there and flying an airplane to gather data and, on the other hand, doing the modeling on a global scale,” says Ahlgrimm. “What I really enjoyed is trying to bridge that gap—as wide as it is—and it’s only worked because I’ve had really good people helping me on either side.”
Keep up with the Atmospheric Observer
Updates on ARM news, events, and opportunities delivered to your inbox
ARM User Profile
ARM welcomes users from all institutions and nations. A free ARM user account is needed to access ARM data.