Let’s unleash the network judgment: A self-supervised approach for cloud image analysis
Submitter
Ferrier, Nicola — Argonne National Laboratory
Collis, Scott Matthew
— Argonne National Laboratory
Area of Research
Cloud Distributions/Characterizations
Journal Reference
Dematties D, B Raut, S Park, R Jackson, S Shahkarami, Y Kim, R Sankaran, P Beckman, S Collis, and N Ferrier. 2023. "Let’s Unleash the Network Judgment: A Self-Supervised Approach for Cloud Image Analysis." Artificial Intelligence for the Earth Systems, 2(2), 10.1175/AIES-D-22-0063.1. ONLINE.
Science
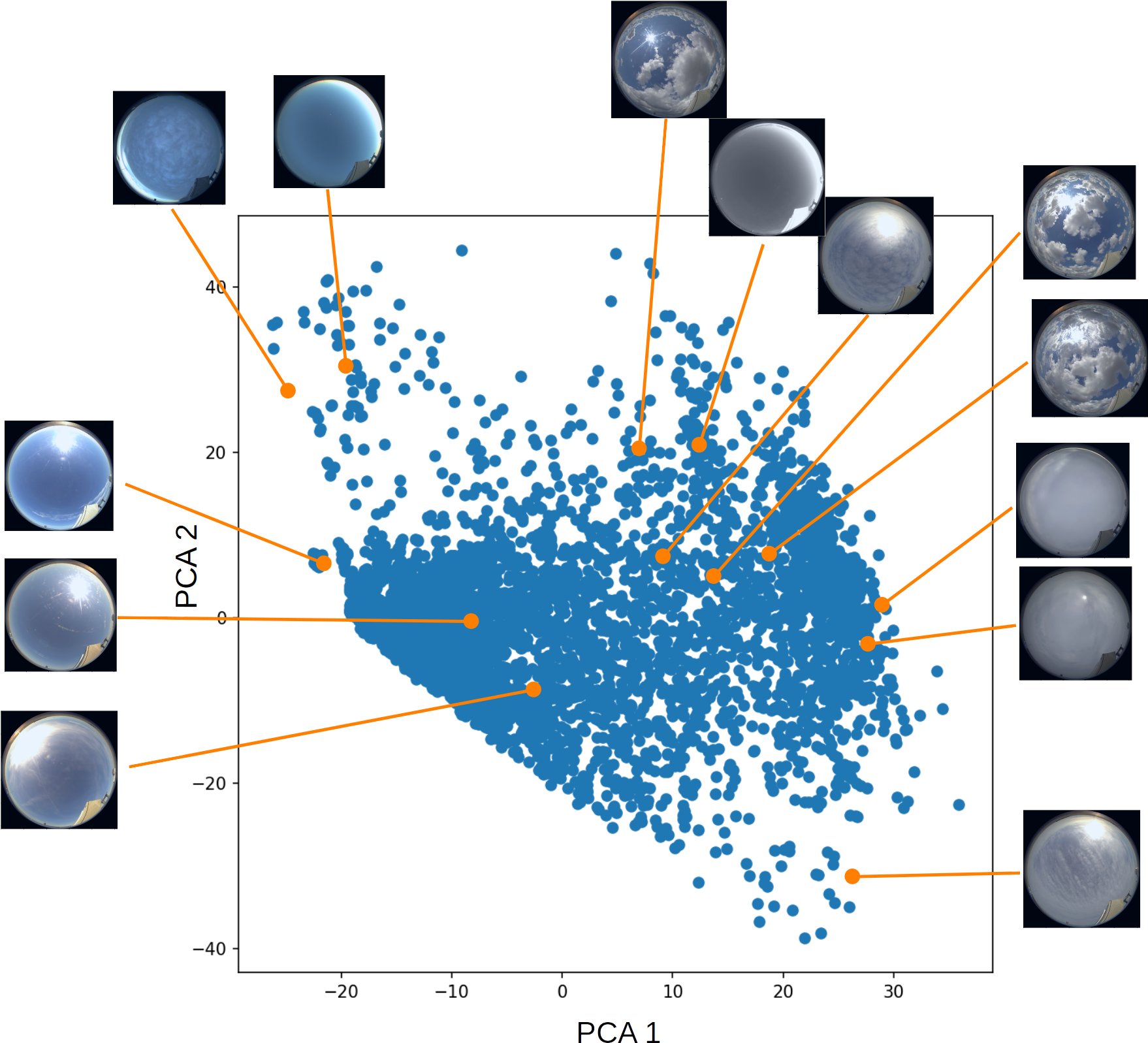
Figure 2. Output feature map from DINO’s inference process. For visualization purposes, we used PCA to bring the 384 dimensions of the
output feature vectors from the model to the two principal components and plotted a subsample with 5,000 points in this chart. From simple pretext
tasks the network is able to acquire feature vectors organized in the space by different properties. From journal.
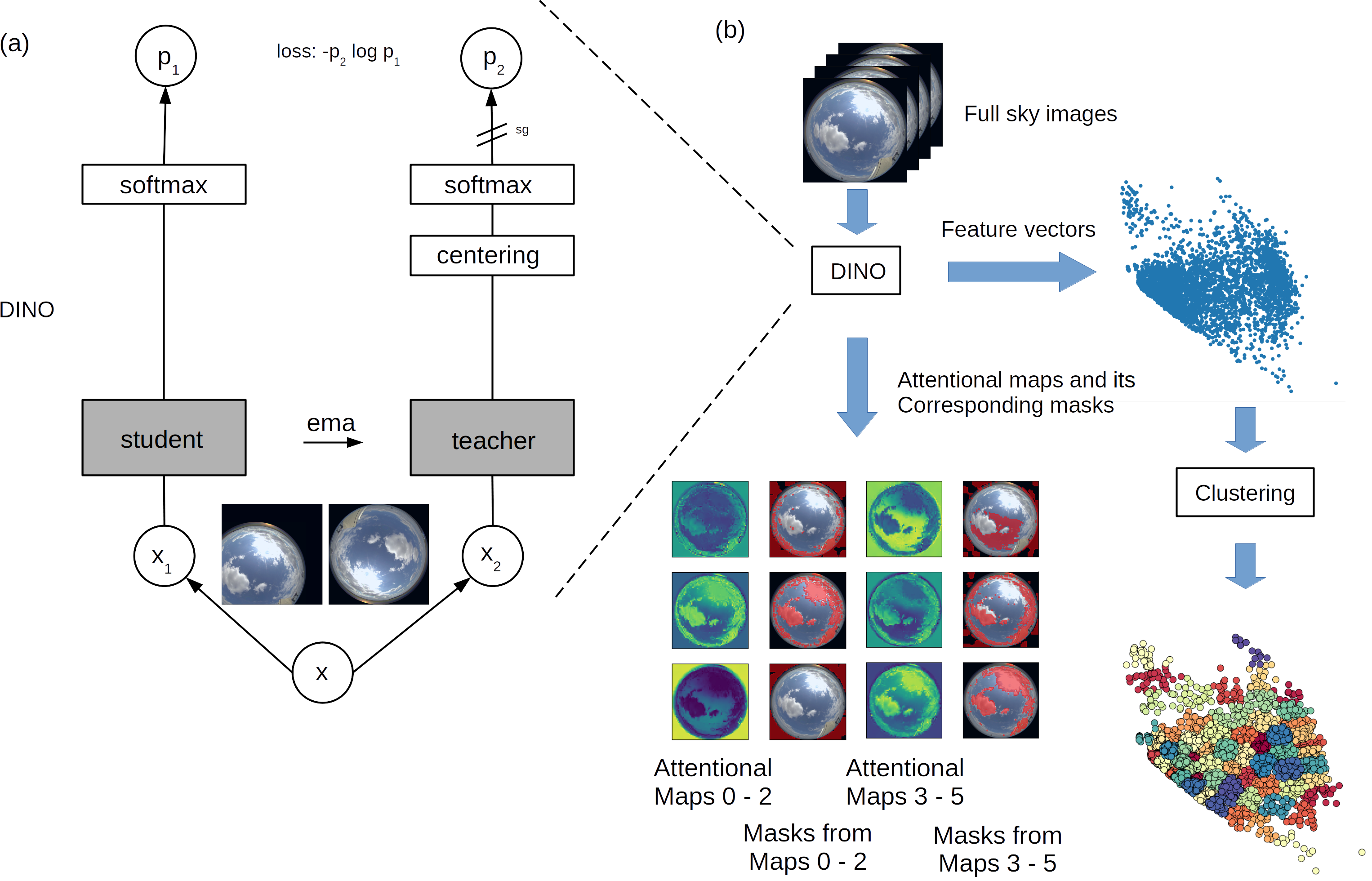
Figure 1. Experimentation profile. (a) A joint embedding model architecture of DINO (DIstillaion NO labels) by Caron et al. (2021b). (b) Once trained, the feature vectors returned by DINO’s inference process are clustered using different techniques, such as SOMs and k-means. Because DINO distributes feature vectors corresponding to different image properties in different zones of the outputs, different clusters will represent images with different visual properties. We use the attentional maps from the ViT in DINO to generate unsupervised segmentation of clouds. From journal.
We present the application of a new self-supervised learning approach to autonomously extract relevant features from sky images captured by ground-based cameras, for the classification and segmentation of clouds.
Impact
We present the application of a novel artificial intelligence approach to autonomously extract relevant features from sky images, for the characterization of atmospheric conditions. Unlike previous strategies, this novel approach requires reduced human intervention, suggesting a new path for cloud characterization.
Summary
Accurate cloud type identification and coverage analysis are crucial in understanding the Earth’s radiative budget. Traditional computer vision methods rely on low-level visual features of clouds for estimating cloud coverage or sky conditions. Several handcrafted approaches have been proposed; however, scope for improvement still exists. Newer deep neural networks (DNNs) have demonstrated superior performance for cloud segmentation and categorization. These methods, however, need expert engineering intervention in the preprocessing steps—in the traditional methods—or human assistance in assigning cloud or clear-sky labels to a pixel for training DNNs. Such human mediation imposes considerable time and labor costs. We present the application of a new self-supervised learning approach to autonomously extract relevant features from sky images captured by ground-based cameras, for the classification and segmentation of clouds. We evaluate a joint embedding architecture that uses self-knowledge distillation plus regularization. We use two data sets to demonstrate the network’s ability to classify and segment sky images—one with ∼ 85,000 images collected from our ground-based camera and another with 400 labeled images from the WSISEG database. We find that this approach can discriminate full-sky images based on cloud coverage, diurnal variation, and cloud base height. Furthermore, it semantically segments the cloud areas without labels. The approach shows competitive performance in all tested tasks, suggesting a new alternative for cloud characterization.
Keep up with the Atmospheric Observer
Updates on ARM news, events, and opportunities delivered to your inbox
ARM User Profile
ARM welcomes users from all institutions and nations. A free ARM user account is needed to access ARM data.