New insights on the prevalence of drizzle in marine stratocumulus clouds
Submitter
Zhu, Zeen — Brookhaven National Laboratory
Kollias, Pavlos — Stony Brook University
Area of Research
Cloud Processes
Journal Reference
ZHU Z, P Kollias, E Luke, and F Yang. 2022. "New insights on the prevalence of drizzle in marine stratocumulus clouds based on a machine learning algorithm applied to radar Doppler spectra." Atmospheric Chemistry and Physics, 22(11), 10.5194/acp-22-7405-2022.
Science
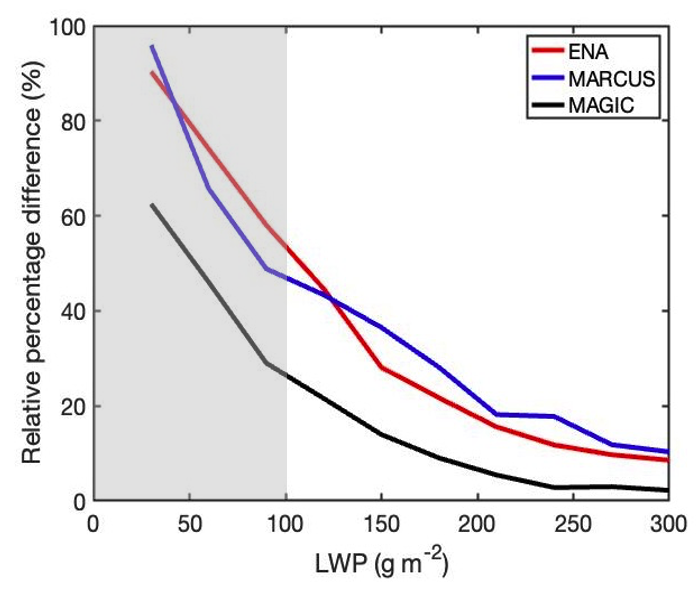
Figure 1. Significant differences are found in drizzle occurrence compared to a traditional methodology. The figure shows the relative percentage difference in drizzle occurrence between the traditional and new detection methods as a function of liquid water path (LWP) for ARM observational campaigns at ENA, MARCUS, and MAGIC (see legend). These results indicate that drizzle is far more ubiquitous in stratocumulus clouds than previously recognized and that the drizzle formation is regime dependent. Spaceborne radar (i.e., CloudSat) observations used to generate precipitation climatologies have low sensitivity in the light precipitation region, which is indicated by the shaded area. Our results have sufficient sensitivity and resolution to provide an alternative view that challenges the conventional light precipitation climatology that may be used to improve the parameterization of warm rain in GCMs. Image from author.
Detecting drizzle embryos is challenging for ground-based remote-sensing observations, which impedes our understanding of the precipitation process in marine stratocumulus clouds. A machine-learning-based approach is developed using unique radar Doppler spectra observations to identify the early stage of drizzle drops.
Impact
Our results demonstrate that drizzle is far more frequent than previously recognized by traditional methods. The method also provides essential information on light precipitation that challenges the detection limits of satellite-borne observations used in precipitation climatologies for global climate model (GCM) evaluation.
Summary
Radar reflectivity from millimeter-wavelength radar is commonly used for drizzle detection, but it is unable to identify weak drizzle signals. Conversely, Doppler skewness—a measure of Doppler spectral symmetry—has proven to be a more sensitive quantity for the detection of drizzle embryos. Here, small drizzle droplets are detected by a newly developed machine-learning technique from unique drizzle retrievals based on radar reflectivity and skewness from millimeter-wavelength radars operated by the Atmospheric Radiation Measurement (ARM) user facility. The drizzle detection algorithm is evaluated by aircraft in situ measurements and then applied to ARM observational campaigns at three different sites (Eastern North Atlantic [ENA], Measurements of Aerosols, Radiation, and Clouds over the Southern Ocean [MARCUS], and Marine ARM GPCI Investigation of Clouds [MAGIC]) to investigate drizzle occurrence in marine stratocumulus clouds. Results show that drizzle is far more ubiquitous than previously recognized, and that the traditional approach significantly underestimates the drizzle occurrence, especially in thin clouds with liquid water path lower than 50 g m-2. Drizzle occurrence in marine boundary-layer clouds differs among the three ARM campaigns, indicating that drizzle formation and distribution is regime dependent, controlled by microphysical and dynamical processes in the local region. Further, spaceborne radar (i.e., CloudSat) observations used to generate precipitation climatologies have low sensitivity in the light precipitation region. The new method provides essential information in this region to challenge the conventional light precipitation climatology and can be used to improve the warm rain parameterization in GCMs.
Keep up with the Atmospheric Observer
Updates on ARM news, events, and opportunities delivered to your inbox
ARM User Profile
ARM welcomes users from all institutions and nations. A free ARM user account is needed to access ARM data.