Entrainment rates in cumulus clouds during GoAmazon 2014/15
Submitter
Eissner, Jordan — University of Kansas
Mechem, David B.
— University of Kansas
Area of Research
Cloud Processes
Journal Reference
Eissner J, D Mechem, M Jensen, and S Giangrande. 2021. "Factors Governing Cloud Growth and Entrainment Rates in Shallow Cumulus and Cumulus Congestus during GoAmazon2014/5." Journal of Geophysical Research: Atmospheres, 126(12), e2021JD034722, 10.1029/2021JD034722.
Science
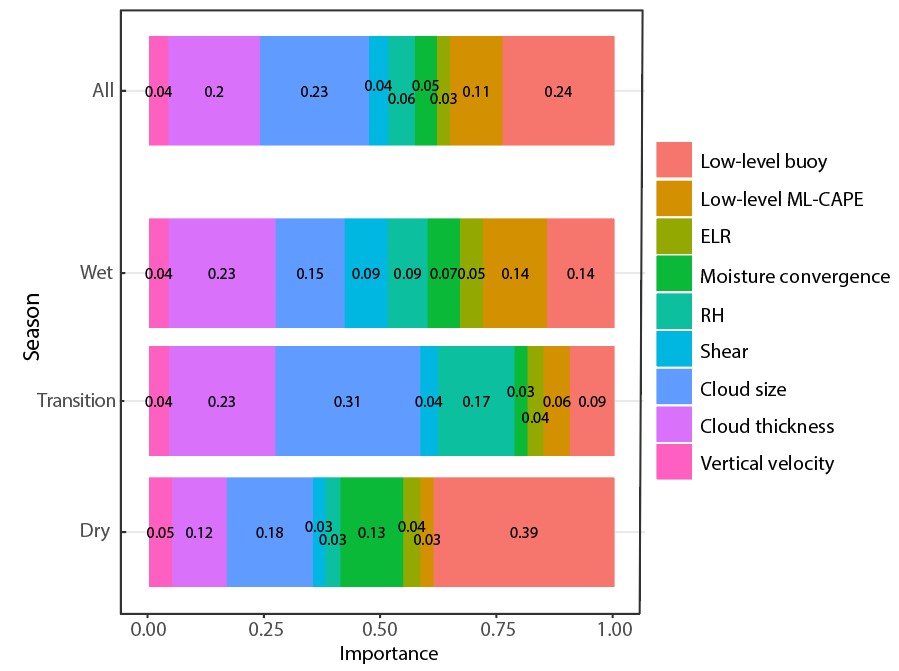
Figure 2. The random forest results of the relative importance of each explanatory entrainment variable for all seasons and in each season. From journal.
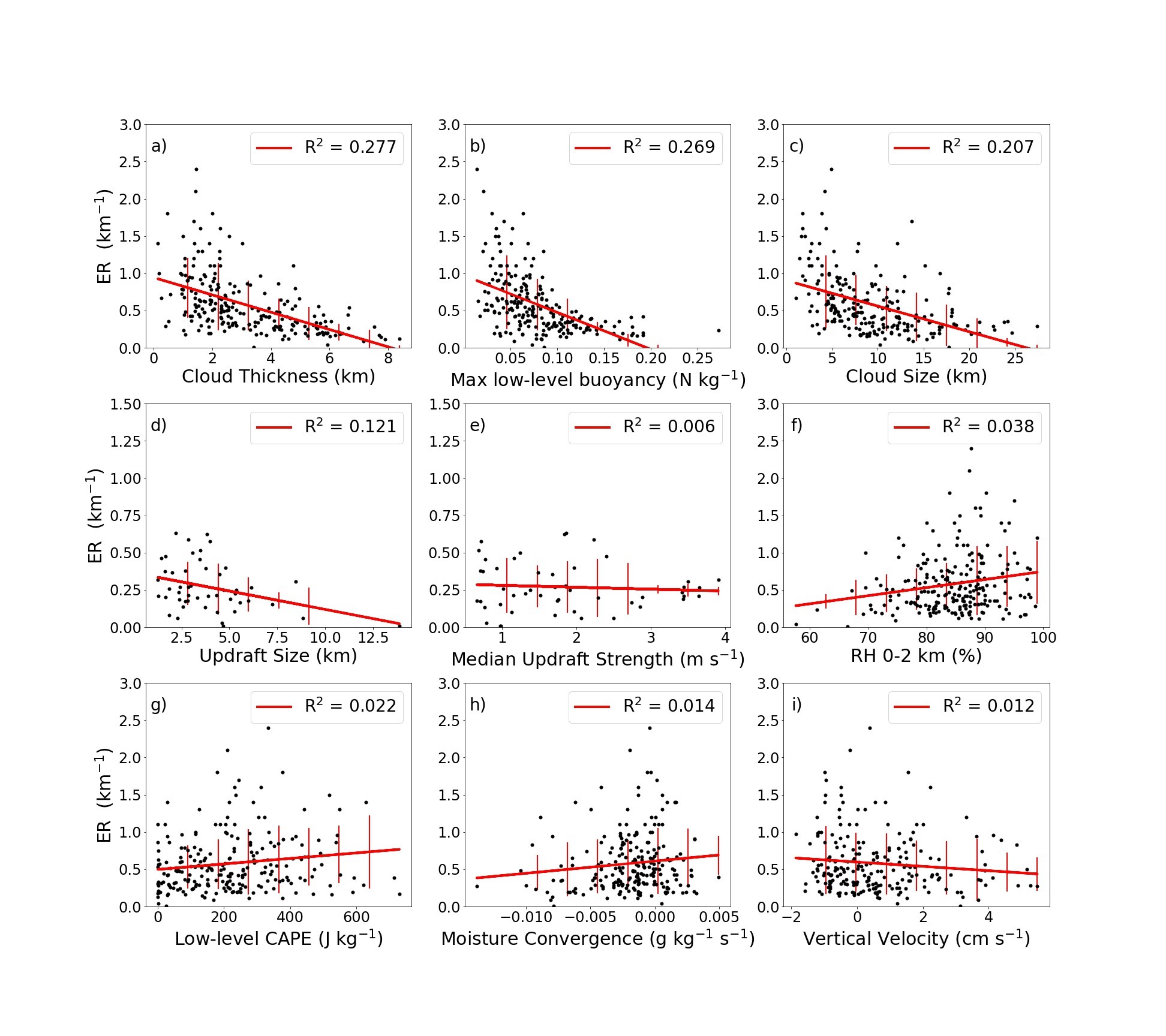
Figure 1. Entrainment rate versus several environmental and cloud variables. The error bars represent the standard deviation of entrainment in appropriately sized bins. From journal.
Entrainment — the mixing between cloud and environmental air outside of clouds — is crucial in determining cloud properties and life cycle. Unfortunately, entrainment is difficult to measure and may be a large source of error in how clouds are represented in models. We use a combination of ground-based remote sensing and radiosondes during the U.S. Department of Energy’s Atmospheric Radiation Measurement (ARM) Green Ocean Amazon (GoAmazon 2014/15) field campaign to estimate entrainment rates in shallow cumulus and cumulus congestus clouds.
Impact
In Earth System Models (ESMs), small-scale cloud processes like entrainment are not resolved and require introducing assumptions about how those processes work. We use the observational entrainment rates we found to evaluate several common approaches for representing entrainment in ESMs. Our observations provide mixed support for these entrainment approximations, including a moderate, negative relationship between entrainment rate and cloud thickness, cloud size, and low-level buoyancy. We also provide an estimate of the uncertainty in our entrainment rates based on uncertainties in the quantities used to estimate the entrainment rate (cloud-top height, and temperature and moisture profiles).
Summary
Much of the uncertainty in ESM climate projections can be attributed to details about how cumulus convection is represented (i.e., parameterizations), with entrainment rate potentially being a large source of uncertainty. Though entrainment has been extensively studied using numerical approaches such as large-eddy simulation (LES), observational techniques to estimate entrainment rates are limited because of the challenge of deploying an instrument suite capable of jointly sampling environmental thermodynamics and cloud properties at high spatial and temporal resolution. The observations taken during the GoAmazon 2014/15 field campaign provide a sufficient sampling of cloud and environmental properties to allow us to estimate entrainment rates using a simple entraining plume model. Measurements from multiple instruments allow us to develop improved estimates of cloud-top height and thermodynamic profiles and provide rigorous uncertainty estimates.
Relationships between shallow cumulus and congestus entrainment rates and environmental and cloud variables are evaluated using linear regression and a random forest, machine learning approach. Both analyses find that cloud thickness, low-level buoyancy, and cloud size have the largest impacts on the entrainment rate, all with an inverse correlation (Figure 1). The random forest analysis shows a clear seasonal variation in the entrainment relationships that is not obvious from simple linear regression. Entrainment tends to be best correlated with cloud thickness and low-level convective available potential energy (CAPE) in the wet season, buoyancy in the dry season, and relative humidity (RH) during the transition season (Figure 2).
Keep up with the Atmospheric Observer
Updates on ARM news, events, and opportunities delivered to your inbox
ARM User Profile
ARM welcomes users from all institutions and nations. A free ARM user account is needed to access ARM data.